AI in Drug Development: A Potential Revolution
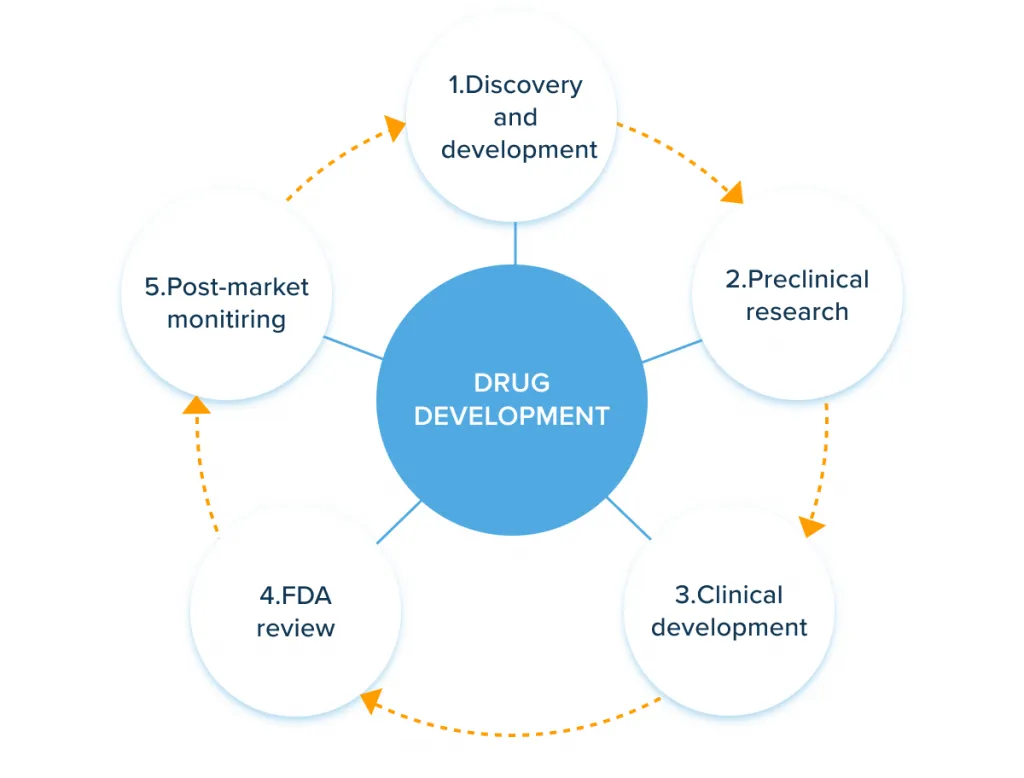
The burgeoning field of artificial intelligence (AI) has ignited a passionate debate in the realm of drug discovery and development. As advancements in AI promise to revolutionize how we identify and create new medications, excitement is tempered by skepticism from industry veterans who caution against overhyping its potential. With notable achievements like AlphaFold, which earned the 2024 Nobel Prize for its groundbreaking work in protein structure prediction, the spotlight is on whether AI can truly mitigate the staggering 90% failure rate of drugs in clinical trials. This exploration delves into the dual-edged nature of AI in pharmaceuticals, examining its capabilities and limitations while questioning if it is a mere trend or a transformative tool in the quest for safer and more effective drugs.
Category | Details |
---|---|
Overview of AI in Drug Development | AI is being explored for drug discovery, aiming to reduce time and costs, but its effectiveness is debated. |
Current Status | AI has led to the discovery of 158 drug candidates between 2010-2022, with 15 reaching clinical trials. |
Time and Cost | Developing a drug currently takes 10-15 years and costs $1-$2 billion. |
Challenges in Drug Development | 90% of drugs fail in clinical trials, often due to safety, efficacy, and dosage issues. |
AI’s Role | AI can assist in identifying drug candidates, predicting toxicity, and selecting trial patients. |
AI Limitations | AI struggles with low-quality datasets and the complexity of drug interactions. |
Future Potential | AI may improve drug selection and reduce costs through innovative strategies. |
New Strategies Proposed | Using machine learning to predict safety, efficacy, and dosage from ignored drug features. |
Phase 0+ Trials | Testing ultra-low doses in patients to better identify effective drugs. |
Conclusion | AI isn’t a magic solution but can help improve the drug development process. |
Understanding AI’s Role in Drug Development
Artificial Intelligence, or AI, is becoming an important part of drug development. It helps researchers find potential new medicines faster and cheaper than before. For example, AI can analyze large amounts of data to identify which compounds might work best in treating specific diseases. This means that instead of testing hundreds of drugs one by one, scientists can use AI to narrow down their choices, saving time and money in the long run.
However, while AI shows promise, it is not a complete solution. Many scientists believe that AI needs to be used carefully. The process of developing drugs is complicated, and just because AI can find good candidates doesn’t mean those drugs will succeed in clinical trials. In fact, many drugs still fail, and researchers need to understand why. So, using AI is just one piece of a much bigger puzzle in drug development.
Challenges of AI in Drug Development
Despite its potential, using AI in drug development comes with challenges. One major issue is that the data used to train AI models is often not very good. For AI to make accurate predictions, it needs high-quality data, but much of the information in drug development is limited or incomplete. This can lead to AI making mistakes or overlooking important details about how a drug might work in humans.
Moreover, many experts in drug development do not have training in AI, which can create communication problems. If scientists do not understand how AI works, they may not use it effectively. This lack of understanding can slow down progress. Therefore, it is vital for researchers to work together and learn how to integrate AI into their drug development processes to overcome these challenges and improve drug discovery.
The Future of AI in Drug Discovery
Looking ahead, the future of AI in drug discovery is exciting but uncertain. AI has the potential to help scientists identify new drug candidates more quickly and effectively. For example, tools like AlphaFold can predict protein structures, which is a crucial step in designing new medicines. If researchers can harness the power of AI correctly, it might lead to breakthroughs that can reduce the high failure rates of drugs in clinical trials.
However, it’s important to remember that AI is not a magic solution. The drug development process is still complex and requires careful planning and testing. Scientists must focus on the underlying reasons why drugs fail, such as safety and efficacy. By combining AI with traditional methods and addressing the core challenges, the pharmaceutical industry can work towards developing more successful drugs in the future.
The Role of AI in Optimizing Clinical Trials
AI is becoming an integral part of optimizing clinical trials, wherein it can analyze vast datasets to identify suitable patient populations. By utilizing machine learning algorithms, researchers can predict which patients are likely to respond positively to specific drugs based on their genetic makeup and medical histories. This targeted approach not only improves patient outcomes but also enhances the efficiency of trials, which traditionally suffer from high dropout rates due to unsuitable candidate selection.
Moreover, AI can help streamline the trial design process itself by simulating different scenarios and outcomes. By modeling various trial parameters, including dosage and treatment combinations, AI tools can identify the most promising strategies before the actual trials begin. This predictive capability allows for better resource allocation and reduces the time spent on unproductive trials, ultimately leading to a higher likelihood of successful drug approval.
Challenges and Limitations of AI in Drug Discovery
Despite its potential, the application of AI in drug discovery is fraught with challenges. One significant limitation is the quality of available data. Many datasets used in training AI models are small and lack the diversity needed to produce reliable predictions. This inadequacy can lead to overfitting, where models perform well on training data but fail to generalize to new, unseen cases, resulting in poor predictions during clinical trials.
Additionally, AI lacks the contextual understanding that human experts possess. While algorithms can process data and identify patterns, they do not inherently grasp the complexities of biological systems. This gap can lead to misinterpretations of data or an incomplete understanding of the mechanisms underlying drug interactions. Addressing these challenges requires a collaborative approach, combining the strengths of AI with the insights of experienced scientists to enhance the drug discovery process.
The Future of AI in Drug Development: Opportunities Ahead
Looking forward, the integration of AI in drug development presents numerous opportunities for innovation. As data collection methods improve and more high-quality datasets become available, AI models will become more robust and accurate. This evolution could lead to the identification of novel drug candidates that might otherwise be overlooked due to the limitations of traditional methods. The ability to analyze complex biological data could transform the landscape of personalized medicine.
Furthermore, advancements in AI technology, such as deep learning and natural language processing, may facilitate more effective collaboration between computational and medicinal scientists. By bridging the gap between data-driven insights and clinical expertise, researchers can develop AI-driven strategies to tackle longstanding challenges in drug development. This collaborative future holds promise for not only improving success rates but also accelerating the time it takes to bring life-saving medications to market.
Ethical Implications of AI in Medicine
The advent of AI in drug discovery raises important ethical considerations that must be addressed. One major concern is the potential for bias in AI algorithms, which can lead to inequitable treatment outcomes. If AI models are trained on data that do not represent diverse populations, they may produce results that are less effective for underrepresented groups. Ensuring that AI systems are fair and inclusive is crucial for ethical drug development.
Additionally, the reliance on AI tools in critical healthcare decisions prompts questions about accountability. In cases where AI-driven predictions lead to adverse outcomes, determining responsibility becomes complex. Establishing clear guidelines and regulatory frameworks will be essential to navigate these ethical dilemmas and ensure that the integration of AI enhances patient safety while promoting trust in the healthcare system.
Frequently Asked Questions
What is artificial intelligence (AI) and how is it used in drug development?
**Artificial Intelligence (AI)** is technology that helps computers learn and make decisions. In drug development, AI is used to find new drugs faster and cheaper by analyzing data.
Why do some scientists think AI in drug development is not a good idea?
Some scientists believe AI in drug development is not effective because most new drugs still fail in trials. They think AI needs to be checked carefully before being fully trusted.
How does AI help speed up the drug discovery process?
AI can analyze huge amounts of data quickly. It helps in finding drug candidates, predicting how safe they are, and determining which patients might respond best to them.
What are the main reasons drugs fail in clinical trials?
Drugs often fail due to three main reasons: **dosage** (how much is given), **safety** (possible side effects), and **efficacy** (how well the drug works).
What is AlphaFold and why is it important?
**AlphaFold** is an AI program that predicts the shapes of proteins, which are crucial for drug design. It won a Nobel Prize for its groundbreaking work in understanding protein structures.
How can AI improve drug testing before they are used on people?
AI can help choose the best drug candidates by predicting their safety and effectiveness in very small doses during early trials, which can save time and money.
What challenges does AI face in drug development?
AI faces challenges like having too little high-quality data and not fully understanding the complex reasons why drugs fail, which can limit its effectiveness in drug development.
Summary
The content explores the potential of artificial intelligence (AI) in drug discovery, emphasizing both its promise and skepticism. While AI tools like AlphaFold demonstrate advancements in predicting protein structures, challenges persist in clinical trial success rates, which remain at 90%. AI’s role in reducing drug development time and cost is acknowledged, yet its effectiveness in improving overall outcomes is questioned. Current strategies focus on addressing dosage, safety, and efficacy through machine learning, suggesting that AI can aid in selecting drug candidates and refining clinical trials. Ultimately, AI may enhance the process but is not a cure-all for drug development challenges.